New publications: Role of Modelling in International Crop Research
“Crop modelling has the potential to significantly contribute to global food and nutrition security,” claim the authors of a recently published paper on the role of modelling in international crop research. “Millions of farmers, and the societies that depend on their production, are relying on us to step up to the plate.”
Among other uses, crop modelling allows for foresight analysis of agricultural systems under global change scenarios and the prediction of potential consequences of food system shocks. New technologies and conceptual breakthroughs have also allowed modelling to contribute to a better understanding of crop performance and yield gaps, improved predictions of pest outbreaks, more efficient irrigation systems and the optimization of planting dates.
While renewed interest in the topic has led in recent years to the development of collaborative initiatives such as the Agricultural Model Intercomparison and Improvement Project (AgMIP) and the CGIAR Platform for Big Data in Agriculture, further investment is needed in order to improve the collection of open access, easy-to-use data available for crop modelling purposes. Strong impact on a global scale will require a wide range of stakeholders – from academia to the private sector – to contribute to the development of large, multi-location datasets.
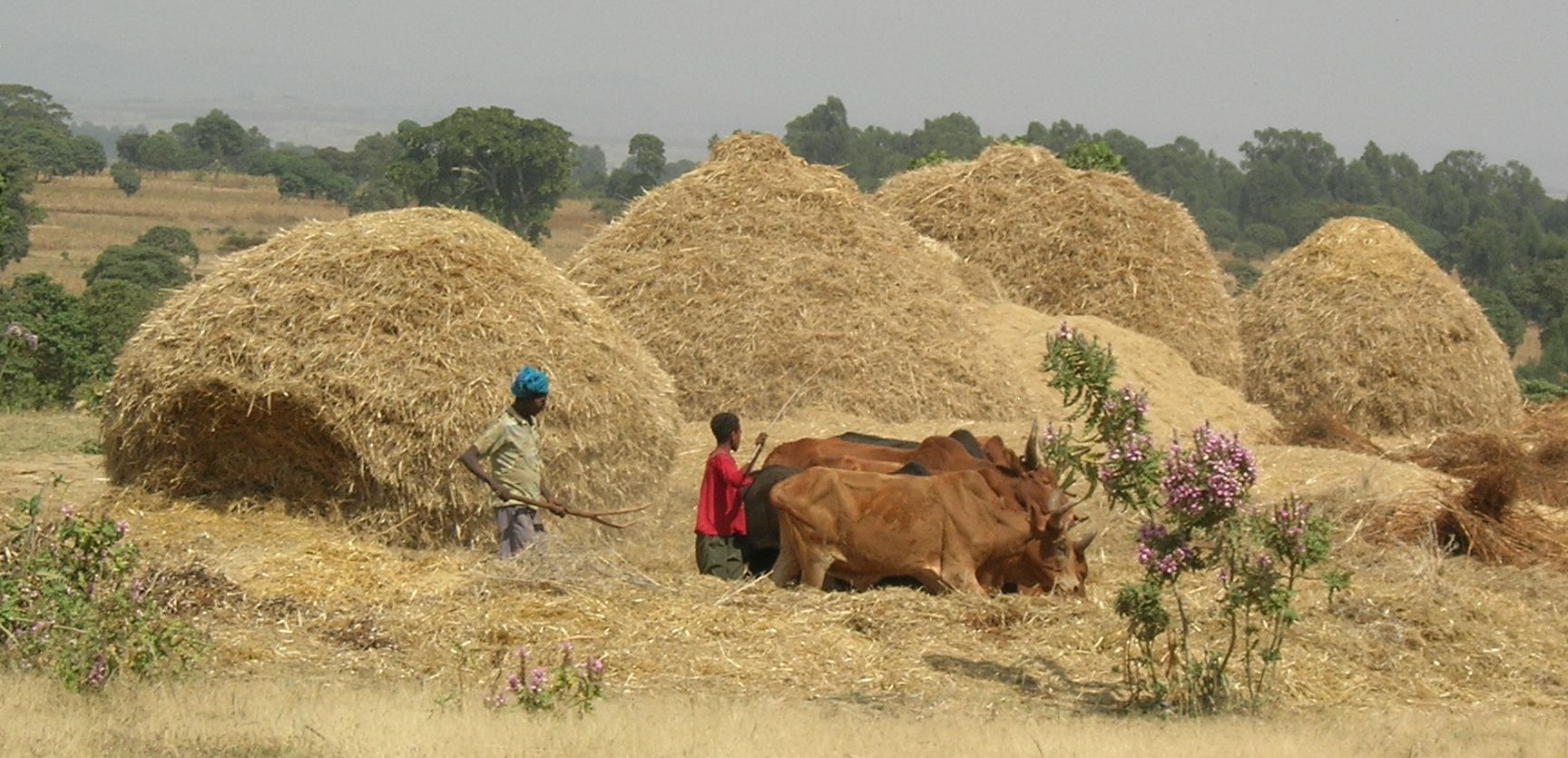
In “Role of Modelling in International Crop Research: Overview and Some Case Studies,” CGIAR researchers outline the history and basic principles of crop modelling, and describe major theoretical advances and their practical applications by international crop research centers. They also highlight the importance of agri-food systems, which they view as key to meeting global development challenges. “The renewed focus on the systems-level has created significant opportunities for modelers to participant in enhancing the impact of science on developments. However, a coherent approach based on principles of transparency, cooperation and innovation is essential to achieving this.”
The authors call for closer interdisciplinary collaboration to better serve the crop research and development communities through the provision of model-based recommendations which could range from government-level policy development to direct crop management support for resource-poor farmers.
Read the full article in Agronomy 2018, Volume 8 (12).
Check out other recent publications by CIMMYT researchers below:
- A framework for priority-setting in climate smart agriculture research. 2018. Thornton, P.K., Whitbread, A., Baedeker, T., Cairns, J.E., Claessens, L., Baethgen, W., Bunn, C., Friedmann, M., Giller, K.E., Herrero, M., Howden, M., Kilcline, K., Nangia, V., Ramirez Villegas, J., Shalander Kumar, West, P.C., Keating, B. In: Agricultural Systems v. 167, p. 161-175.
- Cereal consumption and marketing responses by rural smallholders under rising cereal prices. 2018. Mottaleb, K.A., Rahut, D.B. In: Journal of Agribusiness in Developing and Emerging Economies v. 8, no. 3, p. 461-479.
- Community typology framed by normative climate for agricultural innovation, empowerment, and poverty reduction. 2018. Petesch, P., Feldman, S., Elias, M., Badstue, L.B., Dina Najjar, Rietveld, A., Bullock, R., Kawarazuka, N., Luis, J. In: Journal of Gender, Agriculture and Food Security v. 3, no. 1, p. 131-157.
- Fit for purpose? A review of guides for gender-equitable value chain development. 2018. Stoian, D., Donovan, J.A., Elias, M., Blare, T. In: Development in Practice v. 28, no. 4, p. 494-509.
- Gendered aspirations and occupations among rural youth, in agriculture and beyond: a cross-regional perspective. 2018. Elias, M., Netsayi Mudege, Lopez, D.E., Dina Najjar, Kandiwa, V., Luis, J., Jummai Yila, Amare Tegbaru, Gaya Ibrahim, Badstue, L.B., Njuguna-Mungai, E., Abderahim Bentaibi. In: Journal of Gender, Agriculture and Food Security v. 3, no. 1, p. 82-107.
- Genome-wide association study reveals novel genomic regions for grain yield and yield-related traits in drought-stressed synthetic hexaploid wheat. 2018. Bhatta, M.R., Morgounov, A.I., Belamkar, V., Baenziger, P.S. In: International Journal of Molecular Sciences v. 19, no. 10, art. 3011.
- Identificacion de areas potenciales en Mexico para la intervencion con maiz biofortificado con zinc = Identification of potential areas in Mexico for intervention with biofortified high-zinc maize. 2018. Ramirez-Jaspeado, R., Palacios-Rojas, N., Salomon, P., Donnet, M.L. In: Revista Fitotecnia Mexicana v. 4, no. 3, p. 327 – 337.
- Impact of climate-change risk-coping strategies on livestock productivity and household welfare: empirical evidence from Pakistan. 2018. Rahut, D.B., Ali, A. In: Heliyon v. 4, no. 10, art. e00797.
- Impact of conservation agriculture on soil physical properties in rice-wheat system of eastern indo-gangetic plains. 2018. Kumar, V., Kumar, M., Singh, S.K., Jat, R.K. In: Journal of Animal and Plant Sciences v. 28, no. 5, p. 1432-1440.
- Impact of ridge-furrow planting in Pakistan: empirical evidence from the farmer’s field. 2018. Hussain, I., Ali, A., Ansaar Ahmed, Hafiz Nasrullah, Badar ud Din Khokhar, Shahid Iqbal, Azhar Mahmood Aulakh, Atta ullah Khan, Jamil Akhter, Gulzar Ahmed. In: International Journal of Agronomy v. 2018, art. 3798037.
- Introduction to special issue: smallholder value chains as complex adaptive systems. 2018. Orr, A., Donovan, J.A. In: Journal of Agribusiness in Developing and Emerging Economies v. 8, no. 1, p. 2-13.
- Local dynamics of native maize value chains in a peri-urban zone in Mexico: the case of San Juan Atzacualoya in the state of Mexico. 2018. Boue, C., Lopez-Ridaura, S., Rodriguez Sanchez, L.M., Hellin, J. J., Fuentes Ponce, M. In: Journal of Rural Studies v. 64, p. 28-38.
- Local normative climate shaping agency and agricultural livelihoods in sub-Saharan Africa. 2018. Petesch, P., Bullock, R., Feldman, S., Badstue, L.B., Rietveld, A., Bauchspies, W., Kamanzi, A., Amare Tegbaru, Jummai Yila. In: Journal of Gender, Agriculture and Food Security v. 3, no. 1, p. 108-130.
- Maize seed systems in different agro-ecosystems; what works and what does not work for smallholder farmers. 2018. Hoogendoorn, C., Audet-Bélanger, G., Boeber, C., Donnet, M.L., Lweya, K.B., Malik, R., Gildemacher, P. In: Food security v. 10, no. 4, p. 1089–1103.
- Mapping adult plant stem rust resistance in barley accessions Hietpas-5 and GAW-79. 2018. Case, A.J., Bhavani, S., Macharia, G., Pretorius, Z.A., Coetzee, V., Kloppers, F.J., Tyagi, P., Brown-Guedira, G., Steffenson, B.J. In: Theoretical and Applied Genetics v. 131, no. 10, p. 2245–2266.
- Potential for re-emergence of wheat stem rust in the United Kingdom. 2018. Lewis, C.M., Persoons, A., Bebber, D.P., Kigathi, R.N., Maintz, J., Findlay, K., Bueno-Sancho, V., Corredor-Moreno, P., Harrington, S.A., Ngonidzashe Kangara, Berlin, A., Garcia, R., German, S.E., Hanzalova, A., Hodson, D.P., Hovmoller, M.S., Huerta-Espino, J., Imtiaz, M., Mirza, J.I., Justesen, A.F., Niks, R.E., Ali Omrani., Patpour, M., Pretorius, Z.A., Ramin Roohparvar, Hanan Sela, Singh, R.P., Steffenson, B.J., Visser, B., Fenwick, P., Thomas, J., Wulff, B.B.H., Saunders, D.G.O. In: Communications Biology v. 1, art. 13.
- Qualitative, comparative, and collaborative research at large scale: an introduction to GENNOVATE. 2018. Badstue, L.B., Petesch, P., Feldman, S., Prain, G., Elias, M., Kantor, P. In: Journal of Gender, Agriculture and Food Security v. 3, no. 1, p. 1-27.
- Qualitative, comparative, and collaborative research at large scale: the GENNOVATE field methodology. 2018. Petesch, P., Badstue, L.B., Camfield, L., Feldman, S., Prain, G., Kantor, P. In: Journal of Gender, Agriculture and Food Security v. 3, no. 1, p. 28-53.
- Transaction costs, land rental markets, and their impact on youth access to agriculture in Tanzania. 2018. Ricker-Gilbert, J., Chamberlin, J. In: Land Economics v. 94, no. 4, p. 541-555.
- What drives capacity to innovate? Insights from women and men small-scale farmers in Africa, Asia, and Latin America. 2018. Badstue, L.B., Lopez, D.E., Umantseva, A., Williams, G.J., Elias, M., Farnworth, C.R., Rietveld, A., Njuguna-Mungai, E., Luis, J., Dina Najjar., Kandiwa, V. In: Journal of Gender, Agriculture and Food Security v. 3, no. 1, p. 54-81.